In a move that takes AI agents beyond text-based interactions, OpenAI has released new audio models that bring voice capabilities to their rapidly expanding suite of agent tools. These new models mark a turning point for businesses looking to build voice-based AI systems with natural speech patterns and improved accuracy.
The New Audio Toolkit
OpenAI’s latest release includes three key components:
- GPT-4o-transcribe and GPT-4o-mini-transcribe: New speech-to-text models that outperform previous Whisper models, with better handling of accents, noisy environments, and varied speech speeds.
- GPT-4o-mini-tts: A text-to-speech model with an industry-first feature allowing developers to instruct the model on how to speak, not just what to say.
- Integration with the Agents SDK: Tools that make it easy to convert existing text-based agents to voice agents with minimal code changes.
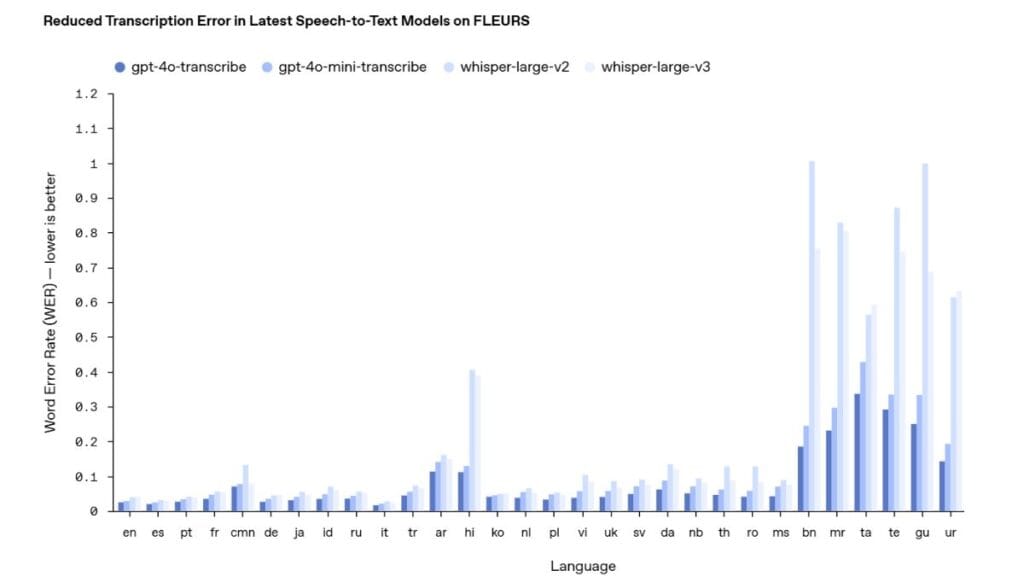
Why These Models Matter for Businesses
The business world has been waiting for voice AI that actually works in real settings. Previous models struggled with accuracy, especially in noisy environments or with speakers who have accents. The new transcription models address these issues head-on.
For customer service teams, this means voice agents that can truly understand callers in busy settings like shopping centers, airports, or crowded homes. For international businesses, it means voice interfaces that work for non-native English speakers without frustration.
The Technical Leap Forward
The new speech-to-text models show significant improvements in Word Error Rate (WER) across languages. This technical metric measures how many words a model gets wrong during transcription.
In practical terms, a lower WER means:
- Fewer misheard customer requests
- Less need for customers to repeat themselves
- More accurate meeting transcripts
- Better data from phone interviews or focus groups
For businesses tracking customer calls, this accuracy improvement translates directly to better data quality and less manual correction.
Tone Control: The Missing Piece in Voice AI
Perhaps the most interesting innovation is the ability to instruct the text-to-speech model on how to speak. This feature solves a major problem with existing voice agents: their flat, emotionless delivery.
Businesses can now create voice agents that:
- Express sympathy when a customer reports a problem
- Sound excited when sharing good news
- Adopt a calm, reassuring tone for sensitive situations
- Speak with authority when giving important instructions
This tone control moves voice agents from obviously robotic to something much closer to human interaction.
Real Implementation: Beyond the Demo
While OpenAI’s demo site (OpenAI.fm) shows off these capabilities, implementing them in a real business setting requires more thought. Here’s what businesses need to consider:
Integration Architecture
Most businesses will benefit from the chained approach OpenAI recommends:
- Speech-to-text captures customer audio
- A text-based LLM processes the request
- Text-to-speech converts the response to audio
This modular design allows for:
- Easier debugging (you can see the text at each stage)
- Mixing models for different needs (e.g., mini models for speed, larger models for accuracy)
- Text logging for compliance and training
Latency Challenges
Voice interfaces have stricter latency requirements than text. Humans notice even small delays in conversation. The new streaming capabilities in the speech-to-text APIs help address this, allowing for processing audio as it arrives rather than waiting for a complete utterance.
Businesses implementing these models should consider:
- Server location relative to users
- Network speed and reliability
- Processing time for complex requests
Cost Considerations
At $0.06 per minute for GPT-4o-transcribe and $0.03 for the mini version, plus $0.01 per minute for text-to-speech, costs add up in high-volume applications. A 5-minute customer service call would cost between $0.20 and $0.35 just for the audio processing, not counting the LLM costs.
Businesses should implement smart timeout policies and consider when to use the mini models vs. the full-sized ones based on accuracy needs.
Industry-Specific Applications
Call Centers
The most obvious application is in call centers, where these models can:
- Transcribe customer calls in real-time
- Flag emotional customers for human intervention
- Provide agents with automated summaries
- Handle routine inquiries without human involvement
EliseAI, an early access partner, reports that implementing these models for housing management communication has increased call resolution rates without human intervention.
Healthcare
In healthcare settings, voice agents can:
- Take patient intake information
- Answer common questions about medications or procedures
- Provide post-care instructions with a calm, reassuring tone
- Transcribe doctor-patient conversations for medical records
The accuracy improvements are critical here, as healthcare information needs to be precisely captured.
Education and Training
Language learning applications can use these models to:
- Create conversation partners for practice
- Provide feedback on pronunciation
- Adjust speaking pace based on learner level
- Switch between different accents or dialects
Corporate training can use them for:
- Role-playing scenarios
- Simulated customer interactions
- Accessible training for visual learners
Retail and E-commerce
Customer-facing retail applications include:
- In-store kiosks that understand questions in noisy environments
- Phone ordering systems with natural conversations
- Product finders that can ask clarifying questions
Technical Implementation Guide
For developers looking to implement these models, here’s a basic workflow:
- Set up audio capture: Ensure clean audio input with noise cancellation
- Implement streaming: Use the new streaming capabilities to process audio continuously
- Choose the right model size: Balance between:
- GPT-4o-transcribe for maximum accuracy
- GPT-4o-mini-transcribe for cost-efficiency
- Design voice instructions: Create a library of tones and styles for different scenarios
- Test in varied environments: Validate performance with different:
- Background noise levels
- Speaker accents
- Speaking rates
- Implement fallbacks: Create graceful handling for when the voice models struggle
- Track and measure: Monitor key metrics like:
- Transcription accuracy
- User satisfaction
- Task completion rates
- Average handling time
The Competition: How OpenAI Stacks Up
OpenAI’s audio models enter a market with established players:
- ElevenLabs: Known for voice cloning capabilities
- Gemini and Nova: Google’s offerings show competitive WER rates in some languages
- AWS Transcribe and Polly: Amazon’s established services for enterprise
How OpenAI’s Models Compare to Existing Solutions
Feature | OpenAI (GPT-4o-Transcribe) | ElevenLabs | Whisper (Older OpenAI Model) |
---|---|---|---|
Word Error Rate (WER) | Low (best for noisy environments) | Medium | Higher WER |
Custom Voice Cloning | No | Yes | No |
Speaker Diarization | No | Yes | No |
Pricing | $0.006 per min | $0.006 per min | Free (Open Source) |
Streaming Support | Yes | No | No |
Best For | Real-time applications | Content creation | Offline transcription |
Where OpenAI appears to lead is in the integration between these audio models and their larger agent ecosystem. The ability to quickly turn text agents into voice agents gives them an edge for businesses already using OpenAI’s tools.
However, OpenAI still lacks custom voice creation, which ElevenLabs currently offers. This remains a gap for brand-specific implementations.
Future Directions
OpenAI has signaled several future developments:
- Custom voices: Allowing businesses to bring their own voices to the platform
- Video capabilities: Expanding beyond audio to full multimodal agents
- Further accuracy improvements: Continued reduction in error rates across languages
For businesses, this roadmap suggests holding off on deep customization of voices if brand-specific voices are important, as this capability should be coming soon.
Practical First Steps for Businesses
For companies looking to implement these new models, here’s a practical approach:
- Audit current customer interactions: Identify which voice touchpoints have the highest volume and simplest structure
- Start with internal tools: Build voice interfaces for employee-facing tools before customer-facing ones
- Test with real audio samples: Use recordings from your actual environment to verify accuracy
- Design for conversation: Create scripts that sound natural when spoken, not just read
- Measure against baselines: Compare accuracy and customer satisfaction against current systems
The most successful implementations will start small, with focused use cases, rather than trying to voice-enable everything at once.
Getting Started Today
The quickest way to test these capabilities is through OpenAI’s demonstration site at OpenAI.fm, where you can try different voice styles and instructions.
For developers ready to build, the updated Agents SDK provides the tools to turn existing text agents into voice agents with minimal code changes. As Yaroslav from OpenAI demonstrated, converting a text agent to voice can be done in as few as nine lines of code.
This accessibility makes voice AI available to businesses of all sizes, not just those with specialized speech technology expertise.
What will your business build with voice?