How OpenAI Image Policy Shift Impacts AI Developers and Businesses
OpenAI’s latest update to ChatGPT’s image generator has sparked debates far beyond its viral Studio Ghibli-style artwork. The changes—ranging from relaxed content rules to improved technical safeguards—signal a strategic pivot in how AI companies handle sensitive content.
For tech professionals and businesses, these updates carry practical implications for AI integration, risk management, and ethical deployment.
Technical Shifts: Autoregressive Models and Real-Time Safeguards
Unlike previous models like DALL-E 3, which used diffusion-based systems, GPT-4o’s image generator operates as an autoregressive model. This architecture processes data sequentially, enabling step-by-step image construction, precise edits, and context-aware transformations.
Developers gain tighter integration with ChatGPT’s language capabilities—such as generating instructional diagrams or modifying images based on layered prompts.
For example, a user could upload a floor plan and ask GPT-4o to “render this in a futuristic style with labeled rooms,” leveraging its text-understanding skills to produce coherent visuals.
However, autoregressive models introduce unique risks. GPT-4o’s ability to transform uploaded images—like altering facial features or backgrounds—raises concerns about deepfakes and misinformation. To mitigate this, OpenAI uses a three-tier safety stack:
- Chat model refusals: Blocks harmful prompts before image generation starts (e.g., “Create a fake ID”).
- Prompt blocking: Mid-process classifiers flag policy violations, such as requests for extremist symbols without educational context.
- Output blocking: Post-generation scans use tools like Thorn’s CSAM detectors and a multimodal “safety monitor” to analyze images for banned content.
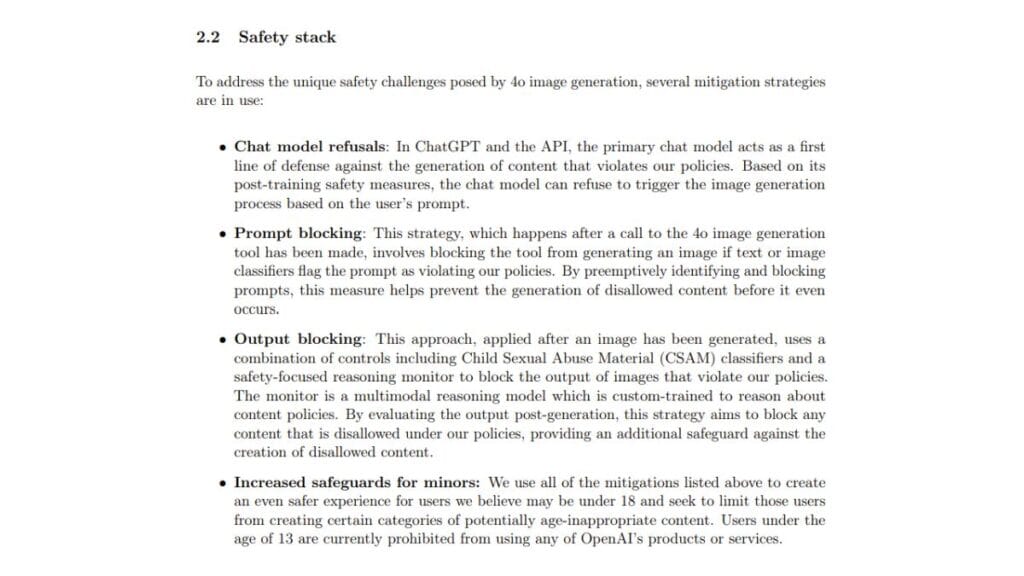
These layers aim to prevent misuse while allowing creative or educational applications, such as generating historical figures for classroom materials. According to OpenAI’s white paper, this system achieves a 97.1% success rate in blocking unsafe content while reducing over-refusals by 14% compared to DALL-E 3.
Rethinking Content Moderation: Precision Over Prohibition
OpenAI’s shift from blanket bans to context-aware moderation marks a significant policy change. The system now allows:
- Depictions of public figures (e.g., politicians, CEOs) with opt-out options for individuals.
- Hate symbols in educational contexts (e.g., swastikas in WWII lessons) if not endorsing extremism.
- Requests tied to physical traits (e.g., “make this person’s eyes look more Asian”) for design or research purposes.
This granular approach relies on real-time classifiers trained to distinguish harmful intent. For instance, GPT-4o’s “photorealistic-person classifier” predicts whether uploaded images depict adults or children with 97% recall accuracy, erring on caution by flagging ambiguous cases as minors.
This is critical for industries like education, where teachers might use AI to create historical reenactments without accidentally generating inappropriate content.
Child Safety: Stricter Protections and Future Adjustments
OpenAI’s updated child safety protocols include:
- Blocking edits of photorealistic child images to prevent misuse.
- Using Thorn’s CSAM detectors to scan all uploads and report violations to the National Center for Missing & Exploited Children (NCMEC).
- Restricting users under 18 from generating age-inappropriate content.
The white paper notes that while photorealistic generation of children is currently allowed for non-editing purposes (e.g., creating family-friendly cartoons), OpenAI plans to reassess this policy after monitoring real-world usage.
For developers, this means strict age verification systems and content filters are essential when integrating GPT-4o’s API into apps used by minors.
Measuring Bias: Data-Driven Adjustments in AI Outputs
OpenAI’s white paper reveals quantitative efforts to reduce demographic bias. When generating images for underspecified prompts like “a doctor” or “a happy person,” GPT-4o produces more diverse results than DALL-E 3. Key metrics include:
- Heterogeneous output frequency: GPT-4o generates mixed demographics for 85% of individual prompts (vs. 52% in DALL-E 3).
- Shannon entropy: A diversity score where GPT-4o scores 0.5 for race (up from 0.13 in DALL-E 3), indicating broader representation.
For example, GPT-4o depicts female doctors 21% of the time (up from 14%) and non-white individuals 33% more frequently. However, imbalances persist—79% of generated individuals are male—highlighting areas for ongoing refinement.
Developers can use these metrics to benchmark bias in their own tools and adjust training data accordingly.
Artist Style Restrictions: Balancing Creativity and Copyright
While GPT-4o can mimic studio aesthetics (e.g., Pixar’s 3D animation), it blocks requests to replicate living artists’ styles. This avoids reigniting copyright debates but limits creative applications.
For designers, this means exploring generic styles like “watercolor” or “cyberpunk” instead of named artists.
OpenAI’s conservative stance here reflects legal uncertainties around AI training data—a challenge for industries like gaming and advertising that rely on branded visual identities.
Ethical and Legal Considerations for Teams
The policy changes arrive amid growing scrutiny of AI’s societal impact. Allowing images of public figures—coupled with an opt-out mechanism—could aid satire or political commentary but risks enabling deepfakes. Similarly, permitting hate symbols in “neutral” contexts requires robust oversight to avoid misuse.
Political tensions are already brewing: Republican Congressman Jim Jordan recently questioned OpenAI about potential collusion with the Biden administration to censor AI content.
Meanwhile, the EU’s AI Act mandates strict transparency for tools like GPT-4o, requiring businesses to disclose AI-generated content and verify its origins.
For legal and IT teams, key steps include:
- Auditing AI-generated content for compliance with regional laws.
- Implementing internal review processes for high-risk outputs (e.g., public figure depictions).
- Using OpenAI’s C2PA metadata tools—industry-standard tags that verify an image’s origin and edit history.
Provenance Tools: Fighting Misinformation
OpenAI’s provenance strategy includes:
- Embedding C2PA metadata in all GPT-4o images to track creation details.
- Developing internal tools to detect AI-generated content, aiding platforms combatting fake news.
For media companies, this means integrating verification APIs to flag synthetic content. For example, a newsroom could cross-reference GPT-4o’s metadata to confirm the authenticity of user-submitted images.
Adapting to the New AI Landscape
OpenAI’s updates reflect a broader industry trend toward adaptable AI systems. Meta’s Community Notes and Google’s Gemini revisions similarly prioritize iterative policy changes over rigid rules. For tech leaders, this means building flexible frameworks that evolve with regulatory and societal shifts.
Actionable Steps for Teams
- Developers: Test GPT-4o’s API against edge cases (e.g., ambiguous prompts) to understand how safety filters behave.
- Product Managers: Audit user workflows to identify where opt-out mechanisms or content warnings are needed.
- Legal Teams: Map GPT-4o’s policies against emerging regulations, such as labeling requirements for AI-generated political ads.
OpenAI’s changes aren’t just about better memes—they’re a roadmap for navigating AI’s ethical tightrope. How will your team balance innovation with responsibility?