OpenAI has launched GPT-4.1, along with smaller siblings GPT-4.1 mini and GPT-4.1 nano. The release marks a significant push toward better coding capabilities in large language models, with OpenAI specifically highlighting improved performance in software engineering tasks.
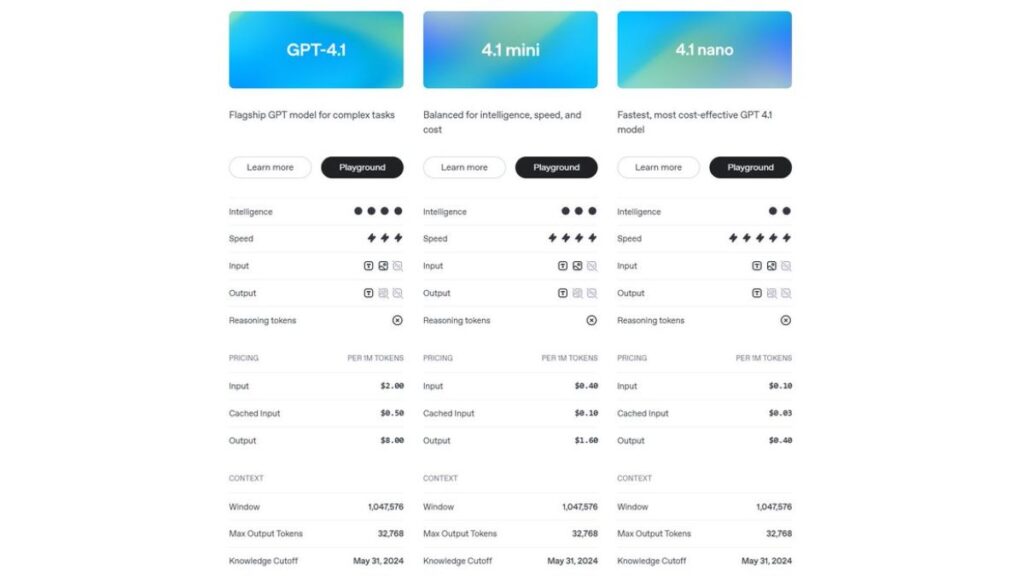
While OpenAI touts impressive benchmark scores, the real question for developers is: how does GPT-4.1 compare to its main competitor, Claude 3.7 Sonnet, when it comes to real-world coding tasks? This article digs deep into both models’ strengths and weaknesses to help you decide which AI assistant best fits your development workflow.
The Models: Key Differences and Similarities
OpenAI’s GPT-4.1 represents a notable advance over GPT-4o with significantly better coding abilities. According to OpenAI, GPT-4.1 scores 54.6% on SWE-bench Verified (a benchmark measuring real-world software engineering skills), which is 21.4% higher than GPT-4o.
Claude 3.7 Sonnet, released by Anthropic in February 2025, scored 62.3% on the same benchmark—higher than GPT-4.1. Both models can handle up to 1 million tokens of context, making them capable of processing entire codebases at once.
The key technical differences between these models impact how they handle different coding scenarios:
GPT-4.1 Strengths:
- Excels at following diff formats accurately
- Better at frontend coding tasks
- Makes fewer extraneous edits to existing code
- More consistent tool usage
- Higher output token limit (32,768 vs 16,384 in GPT-4o)
Claude 3.7 Sonnet Strengths:
- Higher benchmark performance on complex programming tasks
- “Reasoning mode” provides detailed explanations of code generation
- Better handling of edge cases and error conditions
- More thorough step-by-step analysis in debugging scenarios
Real-World Performance Comparison
Benchmark numbers only tell part of the story. Let’s examine how these models perform in typical developer scenarios:
Code Generation and Completion
GPT-4.1 shows marked improvements in generating code snippets that actually work. Its focus on following instructions precisely means it’s less likely to deviate from specific requirements.
However, Claude 3.7 Sonnet has an edge when dealing with complex algorithmic problems. Its reasoning capabilities help it break down difficult tasks into manageable pieces, often producing more robust solutions for problems requiring deep analytical thinking.
For projects using popular frameworks like React, Next.js, or TensorFlow, GPT-4.1 shows better awareness of current practices and conventions. Claude 3.7 Sonnet shines more in situations requiring custom logic or handling unusual edge cases.
Debugging and Problem Solving
When faced with broken code, both models offer different approaches to debugging.
GPT-4.1 is quick to spot syntax errors and common bugs, making it efficient for straightforward debugging tasks. It’s particularly good at identifying issues in frontend code where visual output needs to match specific requirements.
Claude 3.7 Sonnet takes a more methodical approach, often uncovering deeper logical issues that might not be immediately apparent. Its ability to think through potential edge cases makes it valuable for debugging complex systems where multiple components interact.
Working with Existing Codebases
With both models supporting 1 million tokens of context, they can analyze large codebases effectively. However, their approaches differ.
GPT-4.1 is particularly good at making targeted changes to large files while maintaining overall structure. Its improved ability to follow diff formats means you can trust it to modify only what’s needed without introducing unexpected changes elsewhere.
Claude 3.7 Sonnet excels at understanding the architectural implications of code changes. It’s more likely to point out potential ripple effects when modifying core components of a system.
API Integration and Workflow Compatibility
Integrating these models into development workflows requires considering their API features and limitations.
GPT-4.1 offers higher output token limits (32,768) compared to previous models, allowing for more extensive code generation in a single request. It also introduces a 75% prompt caching discount for repeated context, making it more cost-effective when working continuously with the same codebase.
Claude 3.7 Sonnet’s API provides similar capabilities but stands out with its reasoning mode, which gives developers insight into the model’s thought process—particularly useful when evaluating generated code’s reliability.
Cost-Effectiveness Analysis
The economics of using these models varies based on your specific use case:
GPT-4.1 Pricing:
- $2 per million input tokens
- $8 per million output tokens
GPT-4.1 mini:
- $0.40 per million input tokens
- $1.60 per million output tokens
GPT-4.1 nano:
- $0.10 per million input tokens
- $0.40 per million output tokens
While Claude 3.7 Sonnet’s pricing structure is similar to GPT-4.1, the total cost depends largely on how you use the model.
For small, frequent coding tasks, GPT-4.1 nano offers the best value, with speed and cost advantages that make it ideal for code completion and simple fixes. For complex architectural problems or security-critical code reviews, the higher quality results from GPT-4.1 or Claude 3.7 Sonnet likely justify their higher cost.
Code Safety and Security Considerations
Neither model is perfect when it comes to code security. Research has shown that even the best AI models today can introduce security vulnerabilities and bugs.
GPT-4.1 tends to be more cautious about suggesting changes to security-critical code sections but sometimes misses subtle issues. Claude 3.7 Sonnet often provides more detailed security reasoning but can occasionally overcomplicate simple solutions.
A security engineer who evaluated both models noted: “Neither should be trusted blindly for security-critical applications. Both models still require human review, especially for code dealing with authentication, data handling, or input validation.”
Practical Implementation Strategies
To get the most out of these AI coding assistants, consider these implementation strategies:
Best Use Cases for GPT-4.1:
Frontend Development: GPT-4.1 excels at generating and fixing UI components, CSS, and frontend JavaScript. Its improved capabilities with visual elements and layout make it particularly useful for web developers.
Routine Code Generation: For standard patterns like API endpoints, database queries, or unit tests, GPT-4.1 produces reliable results with minimal prompting. The smaller and faster GPT-4.1 mini and nano variants are especially useful here.
Documentation Generation: GPT-4.1 is highly effective at creating clear documentation from existing code, including API references and user guides.
Best Use Cases for Claude 3.7 Sonnet:
Complex Algorithm Design: Claude 3.7 Sonnet’s reasoning capabilities make it better suited for designing complex algorithms or optimization problems that require careful consideration of trade-offs.
System Architecture: When planning how components of a larger system should interact, Claude often provides more thoughtful analysis of potential issues and dependencies.
Bug Hunting in Complex Systems: Claude’s methodical approach to analyzing code makes it particularly good at identifying subtle bugs in complex systems with many interacting parts.
Integration with Development Tools
Both models integrate with popular development environments, but with different strengths:
GPT-4.1’s better handling of diffs makes it ideal for git-based workflows and code review processes. Many developers report success using it with GitHub Copilot and similar tools.
Claude 3.7 Sonnet works well in more exploratory coding scenarios, where you might need to experiment with different approaches to a problem. Its longer reasoning chains help developers understand the rationale behind suggested code.
Use GPT-4.1 integrations for our day-to-day code completion and reviews, but bring in Claude when designing new features or debugging particularly thorny issues.
Looking Forward: How These Models Will Shape Development
The rapid advancement in AI coding capabilities represented by GPT-4.1 and Claude 3.7 Sonnet points to significant changes in how software is developed:
- More Accessible Programming: These models make coding more accessible to people with less technical background, potentially expanding who can contribute to software projects.
- Focus on Higher-Level Tasks: As AI handles more routine coding tasks, human developers can focus on higher-level design decisions and creative problem-solving.
- Changed Skill Requirements: The most valuable developer skills may shift toward prompt engineering, system design, and critical evaluation of AI-generated code.
- Hybrid Development Teams: The most effective teams will likely blend AI capabilities with human oversight, using each for what they do best.
These aren’t just code completion tools anymore. They’re changing how we think about dividing work between humans and machines. Junior devs can now tackle projects that would have required seniors before, and senior devs are free to focus on innovation rather than implementation details.
Making Your Choice: Which Model Is Right for You?
Your choice between GPT-4.1 and Claude 3.7 Sonnet should depend on your specific needs:
Choose GPT-4.1 if:
- You work primarily with popular frameworks and libraries
- You need frequent, smaller code completions and edits
- Frontend development is your focus
- Cost-efficiency for routine tasks is important
- You need to generate a lot of code quickly
Choose Claude 3.7 Sonnet if:
- You work on complex algorithms and systems
- You need detailed reasoning about code decisions
- Finding subtle bugs and edge cases is critical
- You value transparency in how solutions are derived
- Security and robustness are top priorities
Many professional development teams will find value in using both: GPT-4.1 or its smaller variants for day-to-day coding tasks, and Claude 3.7 Sonnet for complex problem-solving and system design.
Final Thoughts
The release of GPT-4.1 marks an important step forward in AI coding assistance, but Claude 3.7 Sonnet remains competitive, particularly for complex programming tasks. Both models represent a significant advance over previous generations and are changing how developers approach their work.
As these models continue to improve, the key challenge for developers isn’t just choosing between them, but learning to work effectively with AI assistance—knowing when to rely on it and when human expertise is still needed.
What’s your experience using these models for coding? Have you found one to be better suited for your specific development needs? Try experimenting with different approaches and share your findings with the wider development community.