The gap between still images and 3D scenes has long been a pain point for creators across multiple fields. Stability AI has now stepped into this space with their new Stable Virtual Camera model, a tool that turns 2D images into dynamic 3D videos with surprising ease.
What Sets Stable Virtual Camera Apart
Stable Virtual Camera isn’t just another AI tool – it’s a shift in how we think about image-to-video conversion. Unlike traditional methods that require complex 3D reconstruction or scene-specific tweaking, this model works with minimal input – as little as a single image.
The system can accept up to 32 input images, but what’s truly impressive is its ability to function with just one. This is a huge leap forward from the usual photogrammetry process, which typically requires dozens of carefully shot photos from different angles.
The model’s real strength lies in its camera control options. Users can define custom paths or choose from 14 preset camera movements, including:
- 360° rotations
- Spiral movements
- Dolly zooms (both in and out)
- Panning in all directions
- Rolling motions
Most AI video generators create content based on text prompts, leaving users with limited control over camera position and movement. Stable Virtual Camera flips this approach by putting camera control front and center.
Technical Deep Dive: How It Works
Stable Virtual Camera uses a multi-view diffusion model trained with a fixed sequence approach. This means it takes a set number of input views and generates a set number of output views during training (M-in, N-out configuration).
The magic happens during the sampling phase. The model uses a two-pass procedure:
- First pass: Generate anchor views that serve as reference points
- Second pass: Render target views in chunks to maintain consistency
This approach allows the model to handle variable input and output lengths (P-in, Q-out) at inference time, making it highly versatile. The model achieves state-of-the-art results in novel view synthesis benchmarks, outperforming previous models like ViewCrafter and CAT3D.
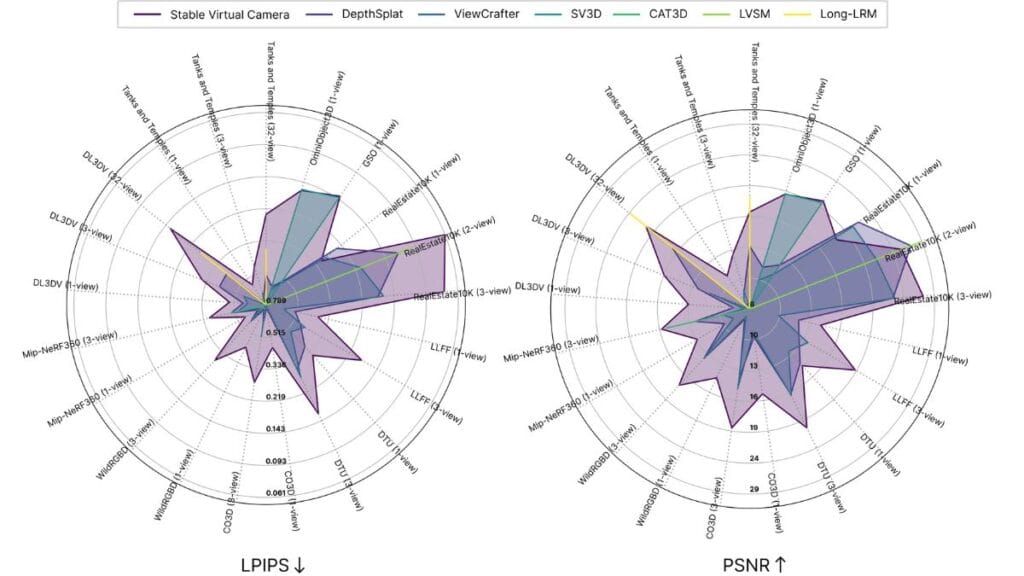
The system excels in both large-viewpoint novel view synthesis (focusing on generation capacity) and small-viewpoint novel view synthesis (prioritizing temporal smoothness). This dual strength is what enables it to create convincing camera movements that maintain object consistency.
Practical Applications Beyond Filmmaking
News coverage has focused on the filmmaking applications, but Stable Virtual Camera has much broader potential:
Game Asset Creation
For indie game developers, this tool could speed up the creation of 3D assets. Rather than modeling everything from scratch, developers could use Stable Virtual Camera to generate basic 3D scenes from concept art or reference photos, then refine them as needed.
While the current non-commercial license limits direct business use, the process demonstrates a workflow that could be replicated using other tools or future commercial versions.
Virtual Reality Content
VR content creators often struggle with the technical hurdles of 3D scene creation. Stable Virtual Camera offers a potential shortcut, allowing the generation of simple 3D environments from flat images.
The ability to create long videos (up to 1,000 frames) with 3D consistency makes it well-suited for creating VR spaces that users can explore.
Architectural Visualization
Architects and designers could use this tool to quickly turn 2D concept drawings into dynamic 3D walkthroughs. This would allow clients to better visualize spaces before construction begins.
The varied camera paths would be particularly useful for creating virtual tours of buildings that don’t yet exist.
E-commerce Product Visualization
Online retailers could transform product photos into 360° views, giving customers a more complete understanding of items before purchase.
The system’s ability to work with multiple aspect ratios (square, portrait, landscape) makes it versatile for various product types and display formats.
Current Limitations and Practical Workarounds
Stable Virtual Camera has some important limitations that users need to be aware of:
Content Restrictions
The model struggles with certain types of content:
- Human figures often show distortion
- Animals tend to look unnatural when animated
- Dynamic textures like water can create artifacts
For best results, users should focus on static scenes, architecture, and objects without complex organic shapes.
Commercial Restrictions
The non-commercial license is a major hurdle for businesses. However, several alternatives exist:
- Similar tools like Luma AI offer commercial licenses for their 3D generation tools
- Traditional photogrammetry software like RealityCapture or Agisoft Metashape remains viable for commercial projects
- The research paper details the model’s approach, potentially allowing companies to develop similar systems in-house
Technical Workarounds
For those looking to use the technology despite its limitations:
- For human figures, consider using a combination approach: generate the environment with Stable Virtual Camera, then add human elements separately
- When working with complex scenes, break them into simpler components and process them individually
- For commercial projects, use the research concepts as inspiration while implementing them in licensed tools
Comparison to Traditional 3D Production Pipelines
To truly understand the impact of Stable Virtual Camera, we need to compare it to traditional 3D workflows:
Traditional Pipeline
- Create or scan 3D models (days to weeks)
- UV unwrap and texture models (days)
- Set up lighting (hours to days)
- Animate camera paths (hours)
- Render final output (hours to days)
Stable Virtual Camera Pipeline
- Select or create input images (minutes)
- Choose camera path (minutes)
- Generate output (minutes to hours, depending on length)
The time savings are substantial, even accounting for the current quality limitations.
The most significant advantage is the removal of technical barriers. Traditional 3D production requires specialized skills in modeling, texturing, lighting, and animation. Stable Virtual Camera makes basic 3D content creation accessible to anyone with a good eye for photography.
Hidden Potential: 3D Asset Extraction
One of the most intriguing possibilities not covered in the mainstream reporting is the potential for 3D asset extraction. While not directly supported by the current release, the model’s ability to understand 3D space from 2D images suggests future versions could output actual 3D models.
This would be a game-changer for:
- Game developers needing to quickly populate virtual worlds
- VR content creators building immersive environments
- Product designers creating digital twins of physical objects
The Twitter comments asking about FBX export highlight this untapped potential. While not currently possible, it points to a clear direction for future development.
The Future of Neural 3D Rendering
Stable Virtual Camera represents an important step in the evolution of neural 3D rendering. It sits at the intersection of several key developments:
- Neural Radiance Fields (NeRF) demonstrated that AI could understand 3D space from 2D images
- Diffusion models proved capable of generating high-quality images with control
- Stable Virtual Camera combines these approaches while adding intuitive camera control
The next logical steps in this evolution would be:
- Improved handling of dynamic elements (humans, animals, water)
- Direct 3D asset export (mesh, textures, etc.)
- Commercial licensing options for business use
Practical Tips for Getting the Best Results
Based on the technical details and limitations, here are some tips for users wanting to experiment with Stable Virtual Camera:
- Choose input images with good lighting and clear subjects
- Avoid complex reflective surfaces when possible
- Start with simple camera paths before attempting more complex movements
- For multi-image inputs, maintain consistent lighting across all images
- Use the GitHub code to experiment with different sampling parameters
How This Fits Into the Broader AI Landscape
Stable Virtual Camera is part of a larger trend of AI tools that bridge the gap between 2D and 3D content creation. Similar technologies include:
- Luma AI’s “Imagine” tool for generating 3D assets from text
- Runway’s Gen-2 model for text-to-video generation
- Google’s Dreambooth for customizing image generation models
What sets Stable Virtual Camera apart is its focus on camera control rather than content generation. While other tools create new content, Stable Virtual Camera transforms existing content into new perspectives.
This approach offers more precision and control, making it particularly valuable for technical fields like architecture, product design, and filmmaking where accuracy matters.
Who Benefits Most From This Technology?
The non-commercial license means this tool is currently best suited for:
- Researchers exploring neural rendering techniques
- Students learning about 3D visualization
- Hobbyists creating personal projects
- Small content creators who can’t afford traditional 3D software
- Professionals testing concepts before committing to full production
For each of these groups, Stable Virtual Camera offers a way to bypass traditional technical barriers to 3D content creation.
Getting Started with Stable Virtual Camera
For those interested in trying the technology:
- Visit the Hugging Face repository to download the model weights
- Check out the GitHub page for code and implementation details
- Read the research paper for technical understanding
- Prepare suitable input images (static scenes work best)
- Experiment with different camera paths to find what works best for your content
The tool’s learning curve is relatively gentle compared to traditional 3D software, making it accessible to newcomers while still offering depth for technical users.
Beyond the Basics: Future Possibilities
Looking ahead, the technology behind Stable Virtual Camera could lead to several exciting developments:
- Real-time applications where users can “move” through still images
- Integration with AR/VR systems for immersive content creation
- Combination with other AI tools for end-to-end content pipelines
- Specialized versions for specific industries (real estate, e-commerce, etc.)
The current research preview is just the beginning of what this approach could achieve.
What makes Stable Virtual Camera truly important isn’t just what it can do today, but what it represents for the future of content creation – a world where the line between 2D and 3D becomes increasingly blurred, and where creating dynamic, immersive content becomes as simple as taking a photograph.
Try it out, push its limits, and think about how it might change your creative workflow. The gap between still image and motion has never been smaller.